Modern synthesis methods have enabled the fabrication of colloids with various different shapes. In addition, plastic waste degrades to nano- and microplastics particles in the environment, also leading to irregular shaped colloidal particles.
These particles, polluting our habitats have unknown implications for our health and habitats. Therefore, understanding self assembly of these non-spherical particles is crucial not only for many applications, like bottom-up fabrication of large ordered systems, but also for understanding the fate of microplastic particles in the aquatic environment.
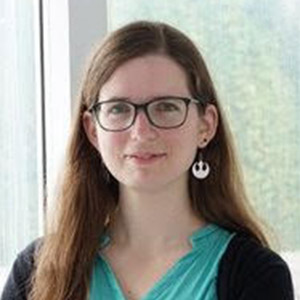
We utilize efficient, large-scale molecular dynamics simulations of coarse-grained models to investigate how non-spherical colloids aggregate. We find that in heterogaggregation of microplastics with other organic matter, smooth round shapes formed compact structures with a large number of neighbors with weak connection between adjacent shapes.
Microplastics with sharper edges and corners aggregated into more fractal structures with fewer neighbors, but with stronger connections. When investigating their behavior under shear, the critical shear rate at which the aggregates break up is much larger for spherical and rounded cube microplastics, and surprisingly, aggregates made of rounded cubes exhibited unexpectedly high stability under shear.
To simulate the non-shperical colloids, we utilized the commonly used composite bead approach to represent the different shapes. We find that this approach is not only expensive as the complexity of the colloidal shape increases, due to inter-body distance calculations, but also cumbersome as it is not always obvious how the composite beads should interact with each other.
Here, I will highlight the use of data-driven methods to accelerate our simulations. We trained neural networks to directly predict energy, forces, and torques between rigid non-spherical particles based on their configuration, bypassing the need for inter-bead distance calculations. This approach can yield significant computational speedup and can be applied to irregular shapes with any pair interaction, given sufficient training data.
Antonia Statt is an Assistant Professor in the Materials Science and Engineering Department at the University of Illinois in Urbana-Champaign since November 2019. Prior to that, she was a postdoctoral fellow at the Princeton Center for Complex Materials, where she worked in the lab of Prof. Athanassios Z. Panagiotopoulos in the CBE Department. She obtained her Ph.D. in the lab of Prof. Kurt Binder in physics at the University of Mainz in Germany. Prof. Statt has received recognition for her research, including the AIChE CoMSEF Young Investigator award, the ACS PRF Doctoral New Investigator Award, and the NSF CAREER award.
Her research is focused on utilizing coarse-grained computational models to further the fundamental understanding of soft matter for applications in energy and medicine. Of special interest are non-equilibrium phenomena like deformation, evaporation, and flow, as well as self-assembly and phase transitions.