A dataset can be represented in different mathematical forms; for example, a micrograph can be represented as an image, as a matrix, as a graph (network), or as an intensity function. These representations are used to perform transformations of the data with the goal of extracting different types of features such as spatial patterns, geometrical patterns, correlations, principal components, gradients of light, and frequencies.
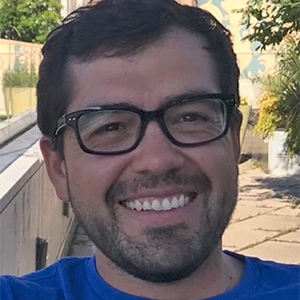
These features contain key information that facilitate visualization and analysis, detection of abnormalities, and construction of predictive models. In this talk, we show how to use representations and transformations in innovative ways to analyze complex datasets arising in flow cytometry, liquid crystals, chemical processes, and molecular dynamics. We show how these tools can be used to design chemical sensors for the detection of contaminants in air and liquid mixtures, to predict reaction rates for acid-catalyzed reactions, to predict material properties from images, and to detect faults.
Victor M. Zavala is the Baldovin-DaPra Professor in the Department of Chemical and Biological Engineering at the University of Wisconsin-Madison and a senior computational mathematician in the Mathematics and Computer Science Division at Argonne National Laboratory. He holds a B.Sc. degree from Universidad Iberoamericana and a Ph.D. degree from Carnegie Mellon University, both in chemical engineering. He is on the editorial board of the Journal of Process Control, Mathematical Programming Computation, and Computers & Chemical Engineering. He is a recipient of NSF and DOE Early Career awards and of the Presidential Early Career Award for Scientists and Engineers (PECASE). His research interests include data science, control, and optimization and applications to energy and environmental systems.